kNN Classification in Python
Visualize scikit-learn's k-Nearest Neighbors (kNN) classification in Python with Plotly.
New to Plotly?
Plotly is a free and open-source graphing library for Python. We recommend you read our Getting Started guide for the latest installation or upgrade instructions, then move on to our Plotly Fundamentals tutorials or dive straight in to some Basic Charts tutorials.
Basic binary classification with kNN¶
This section gets us started with displaying basic binary classification using 2D data. We first show how to display training versus testing data using various marker styles, then demonstrate how to evaluate our classifier's performance on the test split using a continuous color gradient to indicate the model's predicted score.
We will use Scikit-learn for training our model and for loading and splitting data. Scikit-learn is a popular Machine Learning (ML) library that offers various tools for creating and training ML algorithms, feature engineering, data cleaning, and evaluating and testing models. It was designed to be accessible, and to work seamlessly with popular libraries like NumPy and Pandas.
We will train a k-Nearest Neighbors (kNN) classifier. First, the model records the label of each training sample. Then, whenever we give it a new sample, it will look at the k
closest samples from the training set to find the most common label, and assign it to our new sample.
Display training and test splits¶
Using Scikit-learn, we first generate synthetic data that form the shape of a moon. We then split it into a training and testing set. Finally, we display the ground truth labels using a scatter plot.
In the graph, we display all the negative labels as squares, and positive labels as circles. We differentiate the training and test set by adding a dot to the center of test data.
In this example, we will use graph objects, Plotly's low-level API for building figures.
import plotly.graph_objects as go
import numpy as np
from sklearn.datasets import make_moons
from sklearn.model_selection import train_test_split
from sklearn.neighbors import KNeighborsClassifier
# Load and split data
X, y = make_moons(noise=0.3, random_state=0)
X_train, X_test, y_train, y_test = train_test_split(
X, y.astype(str), test_size=0.25, random_state=0)
trace_specs = [
[X_train, y_train, '0', 'Train', 'square'],
[X_train, y_train, '1', 'Train', 'circle'],
[X_test, y_test, '0', 'Test', 'square-dot'],
[X_test, y_test, '1', 'Test', 'circle-dot']
]
fig = go.Figure(data=[
go.Scatter(
x=X[y==label, 0], y=X[y==label, 1],
name=f'{split} Split, Label {label}',
mode='markers', marker_symbol=marker
)
for X, y, label, split, marker in trace_specs
])
fig.update_traces(
marker_size=12, marker_line_width=1.5,
marker_color="lightyellow"
)
fig.show()
Visualize predictions on test split with plotly.express
¶
Now, we train the kNN model on the same training data displayed in the previous graph. Then, we predict the confidence score of the model for each of the data points in the test set. We will use shapes to denote the true labels, and the color will indicate the confidence of the model for assign that score.
In this example, we will use Plotly Express, Plotly's high-level API for building figures. Notice that px.scatter
only require 1 function call to plot both negative and positive labels, and can additionally set a continuous color scale based on the y_score
output by our kNN model.
import plotly.express as px
import numpy as np
from sklearn.datasets import make_moons
from sklearn.model_selection import train_test_split
from sklearn.neighbors import KNeighborsClassifier
# Load and split data
X, y = make_moons(noise=0.3, random_state=0)
X_train, X_test, y_train, y_test = train_test_split(
X, y.astype(str), test_size=0.25, random_state=0)
# Fit the model on training data, predict on test data
clf = KNeighborsClassifier(15)
clf.fit(X_train, y_train)
y_score = clf.predict_proba(X_test)[:, 1]
fig = px.scatter(
X_test, x=0, y=1,
color=y_score, color_continuous_scale='RdBu',
symbol=y_test, symbol_map={'0': 'square-dot', '1': 'circle-dot'},
labels={'symbol': 'label', 'color': 'score of <br>first class'}
)
fig.update_traces(marker_size=12, marker_line_width=1.5)
fig.update_layout(legend_orientation='h')
fig.show()
Probability Estimates with go.Contour
¶
Just like the previous example, we will first train our kNN model on the training set.
Instead of predicting the conference for the test set, we can predict the confidence map for the entire area that wraps around the dimensions of our dataset. To do this, we use np.meshgrid
to create a grid, where the distance between each point is denoted by the mesh_size
variable.
Then, for each of those points, we will use our model to give a confidence score, and plot it with a contour plot.
In this example, we will use graph objects, Plotly's low-level API for building figures.
import plotly.graph_objects as go
import numpy as np
from sklearn.datasets import make_moons
from sklearn.model_selection import train_test_split
from sklearn.neighbors import KNeighborsClassifier
mesh_size = .02
margin = 0.25
# Load and split data
X, y = make_moons(noise=0.3, random_state=0)
X_train, X_test, y_train, y_test = train_test_split(
X, y.astype(str), test_size=0.25, random_state=0)
# Create a mesh grid on which we will run our model
x_min, x_max = X[:, 0].min() - margin, X[:, 0].max() + margin
y_min, y_max = X[:, 1].min() - margin, X[:, 1].max() + margin
xrange = np.arange(x_min, x_max, mesh_size)
yrange = np.arange(y_min, y_max, mesh_size)
xx, yy = np.meshgrid(xrange, yrange)
# Create classifier, run predictions on grid
clf = KNeighborsClassifier(15, weights='uniform')
clf.fit(X, y)
Z = clf.predict_proba(np.c_[xx.ravel(), yy.ravel()])[:, 1]
Z = Z.reshape(xx.shape)
# Plot the figure
fig = go.Figure(data=[
go.Contour(
x=xrange,
y=yrange,
z=Z,
colorscale='RdBu'
)
])
fig.show()
Now, let's try to combine our go.Contour
plot with the first scatter plot of our data points, so that we can visually compare the confidence of our model with the true labels.
import plotly.graph_objects as go
import numpy as np
from sklearn.datasets import make_moons
from sklearn.model_selection import train_test_split
from sklearn.neighbors import KNeighborsClassifier
mesh_size = .02
margin = 0.25
# Load and split data
X, y = make_moons(noise=0.3, random_state=0)
X_train, X_test, y_train, y_test = train_test_split(
X, y.astype(str), test_size=0.25, random_state=0)
# Create a mesh grid on which we will run our model
x_min, x_max = X[:, 0].min() - margin, X[:, 0].max() + margin
y_min, y_max = X[:, 1].min() - margin, X[:, 1].max() + margin
xrange = np.arange(x_min, x_max, mesh_size)
yrange = np.arange(y_min, y_max, mesh_size)
xx, yy = np.meshgrid(xrange, yrange)
# Create classifier, run predictions on grid
clf = KNeighborsClassifier(15, weights='uniform')
clf.fit(X, y)
Z = clf.predict_proba(np.c_[xx.ravel(), yy.ravel()])[:, 1]
Z = Z.reshape(xx.shape)
trace_specs = [
[X_train, y_train, '0', 'Train', 'square'],
[X_train, y_train, '1', 'Train', 'circle'],
[X_test, y_test, '0', 'Test', 'square-dot'],
[X_test, y_test, '1', 'Test', 'circle-dot']
]
fig = go.Figure(data=[
go.Scatter(
x=X[y==label, 0], y=X[y==label, 1],
name=f'{split} Split, Label {label}',
mode='markers', marker_symbol=marker
)
for X, y, label, split, marker in trace_specs
])
fig.update_traces(
marker_size=12, marker_line_width=1.5,
marker_color="lightyellow"
)
fig.add_trace(
go.Contour(
x=xrange,
y=yrange,
z=Z,
showscale=False,
colorscale='RdBu',
opacity=0.4,
name='Score',
hoverinfo='skip'
)
)
fig.show()
k-NN classification in Dash¶
Dash is the best way to build analytical apps in Python using Plotly figures. To run the app below, run pip install dash
, click "Download" to get the code and run python app.py
.
Get started with the official Dash docs and learn how to effortlessly style & deploy apps like this with Dash Enterprise.
Sign up for Dash Club → Free cheat sheets plus updates from Chris Parmer and Adam Schroeder delivered to your inbox every two months. Includes tips and tricks, community apps, and deep dives into the Dash architecture. Join now.
Multi-class prediction confidence with go.Heatmap
¶
It is also possible to visualize the prediction confidence of the model using heatmaps. In this example, you can see how to compute how confident the model is about its prediction at every point in the 2D grid. Here, we define the confidence as the difference between the highest score and the score of the other classes summed, at a certain point.
In this example, we will use Plotly Express, Plotly's high-level API for building figures.
import plotly.express as px
import plotly.graph_objects as go
import numpy as np
from sklearn.neighbors import KNeighborsClassifier
mesh_size = .02
margin = 1
# We will use the iris data, which is included in px
df = px.data.iris()
df_train, df_test = train_test_split(df, test_size=0.25, random_state=0)
X_train = df_train[['sepal_length', 'sepal_width']]
y_train = df_train.species_id
# Create a mesh grid on which we will run our model
l_min, l_max = df.sepal_length.min() - margin, df.sepal_length.max() + margin
w_min, w_max = df.sepal_width.min() - margin, df.sepal_width.max() + margin
lrange = np.arange(l_min, l_max, mesh_size)
wrange = np.arange(w_min, w_max, mesh_size)
ll, ww = np.meshgrid(lrange, wrange)
# Create classifier, run predictions on grid
clf = KNeighborsClassifier(15, weights='distance')
clf.fit(X_train, y_train)
Z = clf.predict(np.c_[ll.ravel(), ww.ravel()])
Z = Z.reshape(ll.shape)
proba = clf.predict_proba(np.c_[ll.ravel(), ww.ravel()])
proba = proba.reshape(ll.shape + (3,))
# Compute the confidence, which is the difference
diff = proba.max(axis=-1) - (proba.sum(axis=-1) - proba.max(axis=-1))
fig = px.scatter(
df_test, x='sepal_length', y='sepal_width',
symbol='species',
symbol_map={
'setosa': 'square-dot',
'versicolor': 'circle-dot',
'virginica': 'diamond-dot'},
)
fig.update_traces(
marker_size=12, marker_line_width=1.5,
marker_color="lightyellow"
)
fig.add_trace(
go.Heatmap(
x=lrange,
y=wrange,
z=diff,
opacity=0.25,
customdata=proba,
colorscale='RdBu',
hovertemplate=(
'sepal length: %{x} <br>'
'sepal width: %{y} <br>'
'p(setosa): %{customdata[0]:.3f}<br>'
'p(versicolor): %{customdata[1]:.3f}<br>'
'p(virginica): %{customdata[2]:.3f}<extra></extra>'
)
)
)
fig.update_layout(
legend_orientation='h',
title='Prediction Confidence on Test Split'
)
fig.show()
Reference¶
Learn more about px
, go.Contour
, and go.Heatmap
here:
- https://plot.ly/python/plotly-express/
- https://plot.ly/python/heatmaps/
- https://plot.ly/python/contour-plots/
This tutorial was inspired by amazing examples from the official scikit-learn docs:
What About Dash?¶
Dash is an open-source framework for building analytical applications, with no Javascript required, and it is tightly integrated with the Plotly graphing library.
Learn about how to install Dash at https://dash.plot.ly/installation.
Everywhere in this page that you see fig.show()
, you can display the same figure in a Dash application by passing it to the figure
argument of the Graph
component from the built-in dash_core_components
package like this:
import plotly.graph_objects as go # or plotly.express as px
fig = go.Figure() # or any Plotly Express function e.g. px.bar(...)
# fig.add_trace( ... )
# fig.update_layout( ... )
from dash import Dash, dcc, html
app = Dash()
app.layout = html.Div([
dcc.Graph(figure=fig)
])
app.run_server(debug=True, use_reloader=False) # Turn off reloader if inside Jupyter
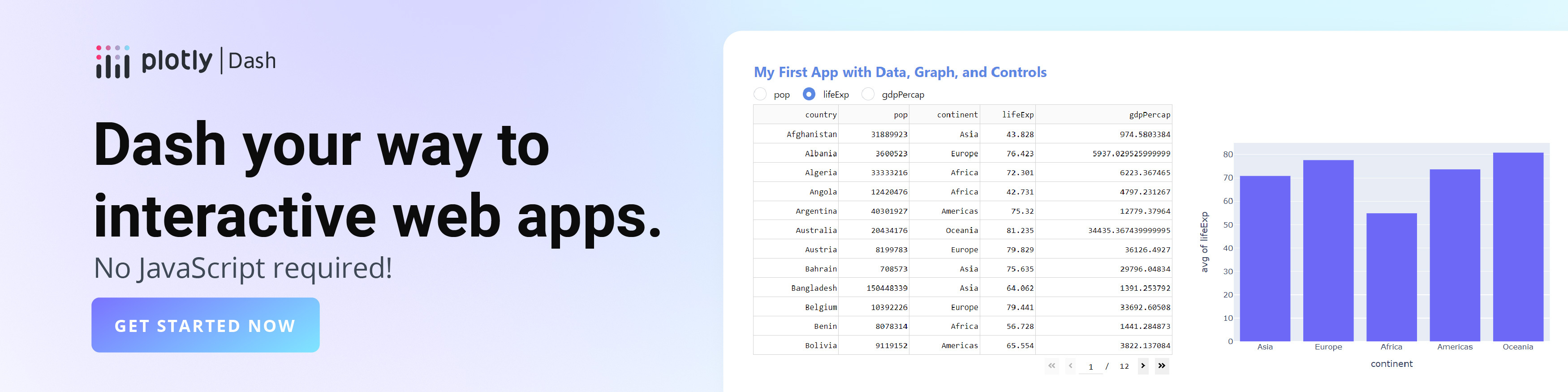